Intelligent Building and Building with (Artificial) Intelligence
Editie: 29 - Artificial Intelligence in the Built Environment
Published on: 23 maart 2022
Building tangibility in the era of virtuality
In the era of digitalization and an increasingly virtual world, the built environment and brick-and-mortar real estate are easily placed among the few tangible things left. Yet, not without reason, the built environment is also being taken over by Artificial Intelligence (AI). While some debate about what role AI could play in this domain has happened in the past, any doubt is now over. Over the last decade, Building Information Modelling (BIM), AI, Internet of Things (IoT) and Construction Tech have been at the forefront of the (technological) developments in the built environment and have redefined the design, construction and operation of buildings and infrastructure. Reducing negative environmental impact, increasing productivity, aiding energy transition, and enabling evidence-based approaches are just a few of the benefits of AI applications demonstrated in research and practice. However, despite the broad agreement that AI is here to stay, the mysticism surrounding the term and its implementations in the built environment still dominates the field.
AI: teaching machines to read between the lines with semantics and statistics
AI is concerned with designing intelligent systems that exhibit characteristics associated with human intelligence, i.e., understanding language, learning, reasoning, etc. (Barr & Feigenbaum, 1981). In other words, AI is a collective term for software applications that approach problem-solving in a way that approximates human intellect. Such applications are enabled by the two main areas constituting AI – statistical (i.e., machine learning, data mining, pattern recognition, probabilistic models) and symbolic (semantic data modelling, formal logic, automated reasoning, linguistics, ontologies) (Hoehndorf & Queralt-Rosinach, 2017)
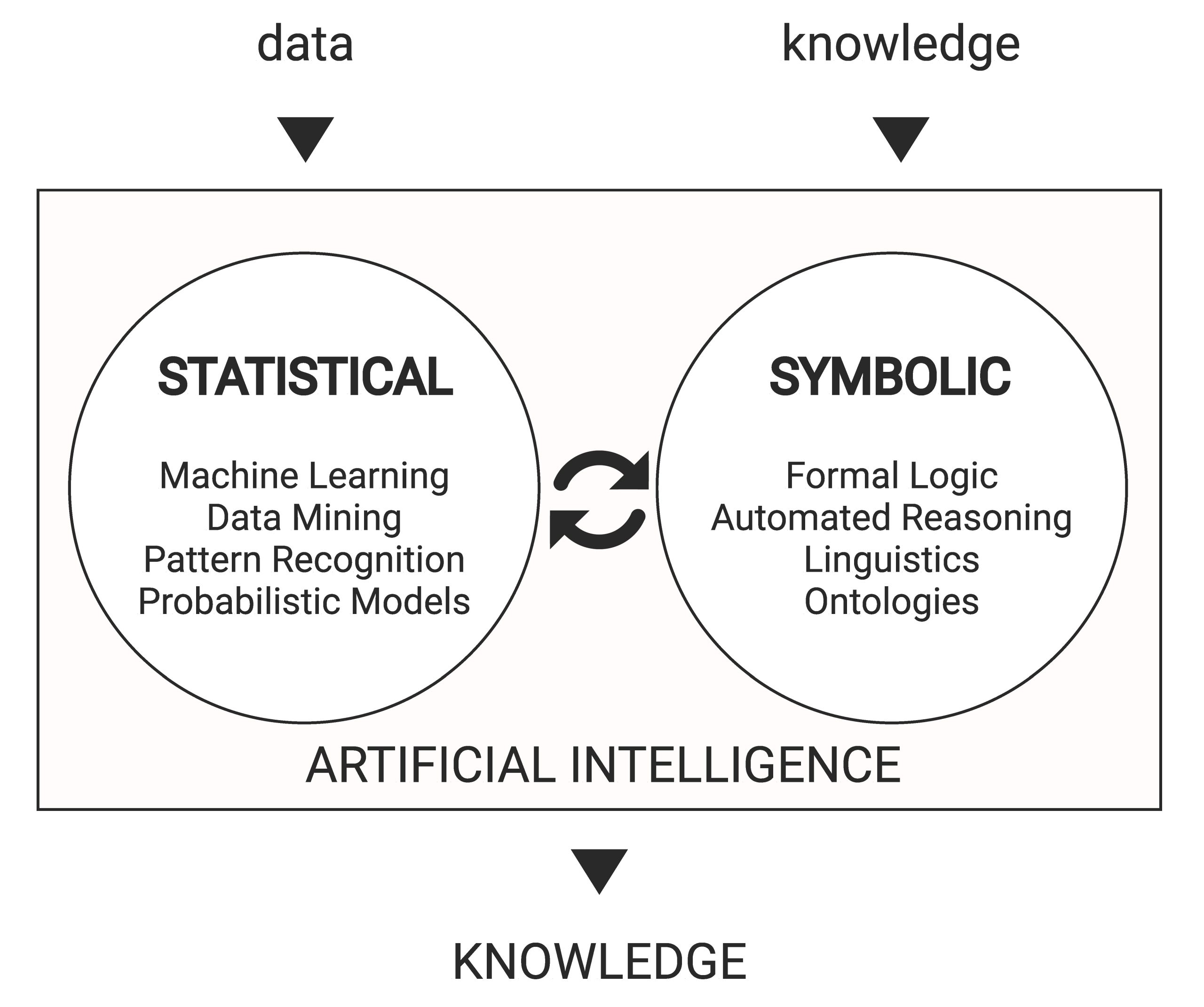
Figure 1. Statistical and symbolic constituents of Artificial Intelligence, based on Hoehndorf & Queralt-Rosinach (2017).
Statistical and symbolic AI approaches have come a long way in processing large volumes of data, finding patterns, establishing relationships in data, predicting future behaviour, explaining phenomena, and emulating human problem-solving (Petrova et al., 2019). Machine learning algorithms efficiently discover novel knowledge in data and constitute a large part of statistical AI (Bishop, 2006). Symbolic AI focuses on human knowledge representation, semantic modelling and disambiguation of data. Symbolic approaches articulate the rich semantic links in data in a machine-readable form, allowing knowledge reuse, inference, and human-like reasoning (Sowa, 2008).
Within the built environment, machine learning aids the discovery of novel insights from data generated from, for instance, sensors and sensor networks. On the other hand, semantic technologies (i.e., knowledge graphs) help represent relations between buildings, locations, spaces, and other heterogeneous data (Petrova et al., 2019). Both approaches have independently received attention in research and practice, as they provide the necessary infrastructure for decision support in different ways.
To harvest the full potential of AI, a reconciliation between statistical and symbolic AI is essential. Symbolic AI can boost machine learning and enhance human decision-making. That enables building more intelligent solutions and trust in the decisions. Furthermore, to demonstrate the value of AI, data should be treated in depth with machine learning algorithms and in breadth, as that allows capturing the evolution of the discovered insights over time. Thus, integrating symbolic and statistical methods can help achieve holistic decision support, which cannot be achieved with any of the approaches alone (Petrova, 2019).
It is important to note that domain expertise, the capability to choose and apply the suitable methods the right way, and accurate data definition are also essential. Moreover, massive data does not automatically guarantee novel insights and understanding of the data (Lausch et al., 2015). Uncovering such insights needs a continuous flow of appropriately treated data to the appropriate analytical mechanisms and stakeholders. So far, most efforts have focused on analysing raw data. Equally important, however, are breaking up isolated data silos, retrieving data from various sources effectively, enabling the interpretation of previously unseen datasets, reusing and integrating heterogeneous data, and making data readable and understandable by machines and humans (Janowicz et al., 2015). In other words, bringing out the value in data using AI requires an infrastructure for analysing data with high-performance algorithms and for publishing, storing, retrieving, reusing, and integrating data, which semantic approaches excel at (Petrova, 2019).
When AI meets the built environment
The affordability of computing power and the advancements in BIM, AI and IoT have created a paradigm shift in design, monitoring and improving the performance of all aspects of the built environment. The richness and availability of data create the perfect conditions for AI to enhance performance, sustainability and efficiency. AI has various implementations in the built environment (Figure 2). They all aim for improving (design) decision-making and building performance; analysis of urban dynamics; prediction of energy use; fault detection and diagnosis in system operation; building energy management; holistic building design; linking and exploring datasets, etc. (Petrova, 2019). Ultimately, all these efforts serve the same purposes – decarbonizing, improving performance, indoor environmental quality, occupant comfort and well-being, safety, cost-efficiency, and productivity. At the same time, reducing resource use, operational costs and risks are equally important targets. AI can also help establish best practices, help humans better identify and understand construction delays and bottlenecks, and predict progress and construction costs more accurately. By utilizing the vast amounts of heterogeneous data, AI can also help identify the fluid criteria of desirable real estate, and identify and track patterns of traffic, space utilization and occupant behaviour.
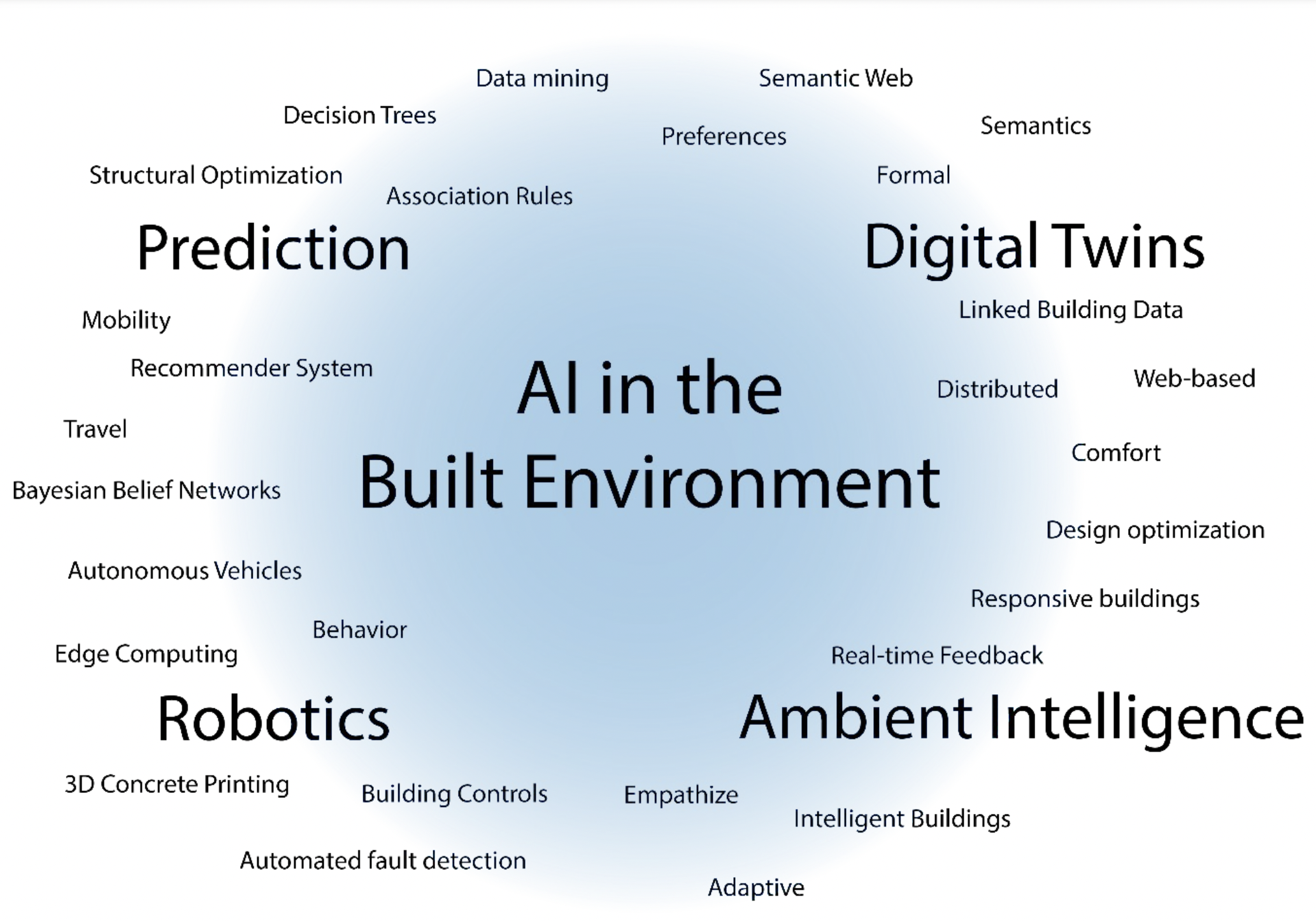
Figure 2. Artificial Intelligence methods and implementations in the built environment (Image courtesy: Department of the Built Environment, Eindhoven University of Technology.
At the building level, the use of AI has a significant impact on both design, construction, and operation. The design stage is associated with a high level of uncertainty and has a tremendous impact on the performance of the buildings in operation. AI has changed the way architects and engineers envision and create the built environment. Machine learning applications are used in parametric design emulation, generative design, design evaluation, simulation, building performance prediction and optimization (Hong et al., 2020). Material and space utilization, acoustic performance, safety, energy efficiency, and occupant well-being are among the main objectives in this regard. Semantic approaches are leveraged for data modelling (i.e., Linked Building Data), solving interoperability challenges, linking data across domains, as well as for logical inferences and proofs in the context of rule checking and building regulation compliance checking (Pauwels et al., 2017). Recent efforts also rely on Natural Language Processing (NLP) for automated compliance checking (Amor & Dimyadi, 2021). Integrating symbolic and statistical AI can enable a feedback loop between building operation and design, and inform decision making in an evidence-based way (Petrova, 2019).
AI is also beneficial in the construction process. Both semantics and machine learning enable advanced cost analysis, construction scheduling and logistics, site monitoring, defect detection, construction waste management and robotic construction (Hong et al., 2020; Boje et al., 2020). Robots, computer vision and LIDAR imaging help detect issues on construction sites before they lead to costly consequences. In addition, digital manufacturing and 3D (concrete) printing also redefine the production aspects of built facilities.
Leveraging operational building data to provide decision support to the right systems and people at the right time has the potential to bridge a major disconnect in building design, construction, and operation. Stakeholders in the built environment face the challenge of operating low energy buildings while balancing high expectations for occupant comfort and well-being. AI enables fault detection and diagnosis in building systems, energy efficiency improvement and post-occupancy evaluation (Hong et al., 2020). Machine learning can be used to predict weather, occupancy, building loads and transform the predictive information into optimization. Research explores machine learning for smart building control, where Model Predictive Control and reinforcement learning-based control take the lead (Hong et al., 2020). Digital Twins are hereby a fundamental concept, as they leverage live data warehouses and enable dynamic simulations and predictive maintenance (Sacks et al., 2020; Boje et al., 2020). State-of-the-art research targets integrating data, robotic technologies and AI to create bi-directional Digital Twins capable of actuation.
Newly emerged areas such as Ambient Intelligence aim to bring intelligence and sensitivity to everyday environments and make them respond to individual user needs and requirements (Monekosso et al., 2009). AI, robotics and IoT transform living spaces into adapting living organisms that understand and empathise with the user (Mohammadi & Hammink, 2015). Finally, AI also influences building retrofit by helping identify building retrofit potential, evaluate energy conservation measures, and characterize buildings (Hong et al. 2020).
Needless to say, AI is also changing the landscape of urban planning, land use, mobility and transportation. Improving urban mobility relies on personal information systems and decision support based on advanced visualization techniques, Digital Twins of cities, buildings and people, as well as machine learning algorithms for predictions and providing personalized advice. Considering the latest advances in transport technology, such as autonomous driving, electric vehicles, and Mobility as a Service, developing models of citizens’ travel behaviour has never been more important (Labee et al., 2022). In that relation, AI is vital for traffic simulations and predictions, demand responsive transport and generating spatial and temporal insights into travel and activities. Furthermore, the interaction of users with buildings, infrastructure and transportation networks is a valuable input to decision support in the context of smart buildings and cities.
All concepts and applications described above intertwine and converge in the Smart City concept. Smart Cities entail a transformation of the buildings and urban environments from a static medium hosting inhabitants to an interactive and intelligent medium that interacts with the occupants and proactively participates in their daily activities. Smart buildings, IoT sensors, actuators, wearables, autonomous vehicles, and Digital Twins build an environment with intelligence on the edge (Khan et al., 2019) that interacts with its users and responds to their needs.
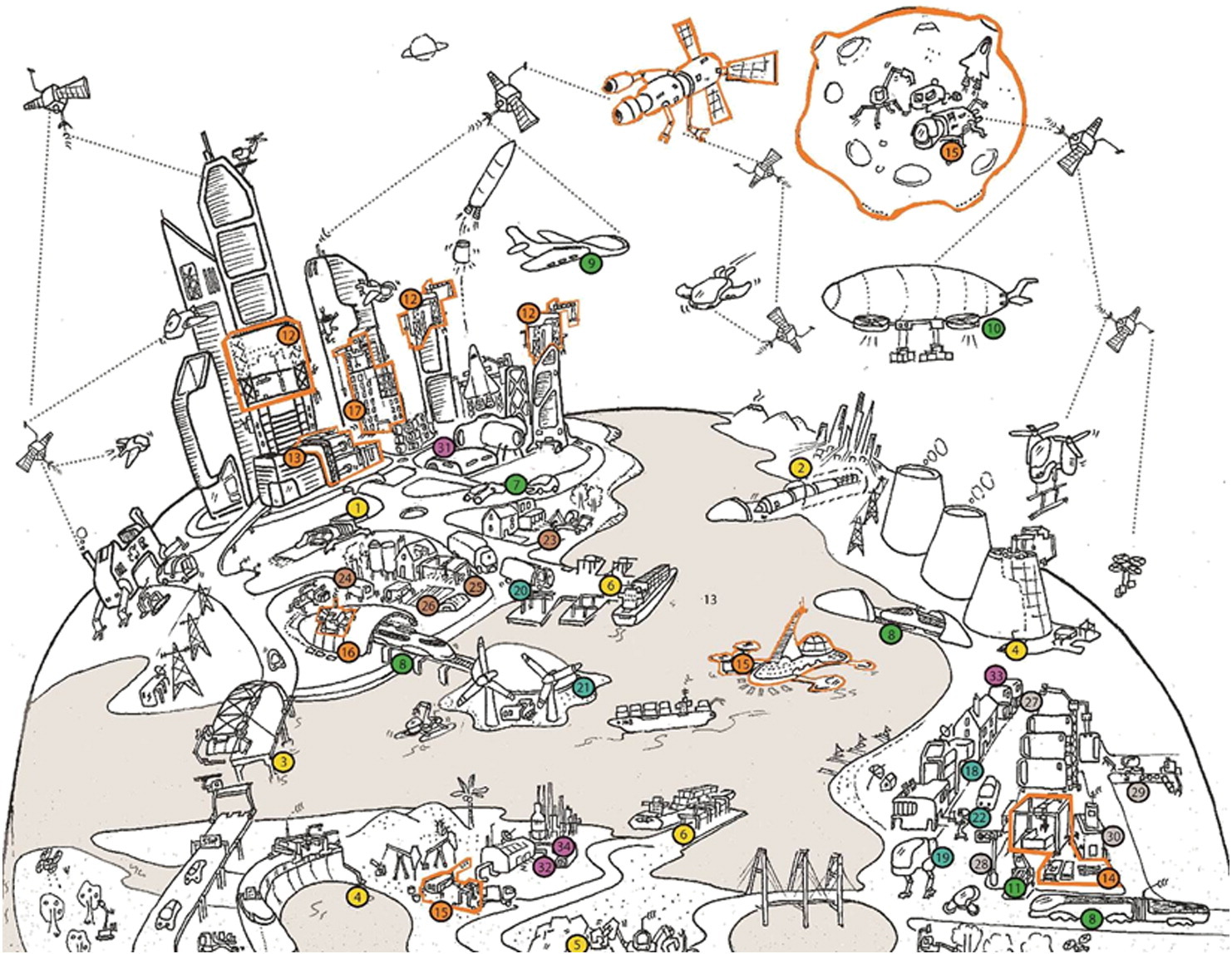
Figure 3. Automation and robot technology becoming ubiquitous and gradually pervading our life on earth as envisioned in Bock (2015).
Property AI or how AI changes the real estate market
Property AI refers to AI in the real estate space, which is also redefined by emerging technologies and data-driven approaches. A typical example is relying on AI to personalize the user experience when searching for real estate. Using customer segmentation data like age, occupation, income, etc., provides the necessary input for machine learning algorithms to profile users and prioritise specific property characteristics. Computer vision-powered systems can also help users find properties with desired characteristics, even if those are not present in the listing metadata. Examples include image classification to detect whether a room has been renovated or whether specific materials and architectural details are present.
Dynamic property valuation is another aspect of real estate, which AI can significantly improve. Traditional property valuation suffers manual processes, lack of consistency and unclarity when it comes to influencing factors. An AI-driven valuation can leverage public records, crime statistics, institutional ratings, and access to various facilities to ensure accuracy, transparency and efficiency. Machine learning algorithms are also rather valuable for identifying the factors that impact property prices (Krämer et al., 2021, Nunez-Tabalez et al., 2013). Finally, intelligent computer systems can also leverage browsing history, session length and other available data to identify the most interested users and feed that information to agents.
Epilogue
The large volumes of data generated throughout the entire building life cycle, combined with the rapid advancement in AI, give unprecedented opportunity to develop advanced and technologically sound and tailored solutions for decision support of the diverse stakeholders in the built environment. However, the ultimate common goal of all stakeholders remains the same – to achieve a sustainable, healthy, high-quality built environment efficiently. State-of-the-art research leverages the latest advancements in AI to amplify human decision-making in an assistive, user-centred, symbiotic, and effective manner. The rest remains to be seen – to what extent is the future of AI human and the future of humans – AI?
References
Amor, R., Dimyadi, J. (2020). The promise of automated compliance checking. Developments in the Built Environment, 5, 100039.
Barr, A. & Feigenbaum, E. (1981). The Handbook of Artificial Intelligence, Los Altos, CA, USA: William Kaufmann, Inc.
Bishop, C. M. (2006). Pattern recognition and machine learning. Cambridge, United Kingdom: Springer.
Boje, C., Guerriero, A., Kubicki, S., & Rezgui, Y. (2020). Towards a semantic Construction Digital Twin: Directions for future research. Automation in Construction, 114, 103179.
Hoehndorf, R. & Queralt-Rosinach, N. (2017). Data Science and symbolic AI: Synergies, challenges and opportunities. Data Science, 1, 27–38.
Hong, T., Wang, Z., Luo, X. & Zhang, W. (2020). State-of-the-art on research and applications of machine learning in the building life cycle. Energy and Buildings, 212, 109831.
Janowicz, K., van Harmelen, F., Hendler, J. & Hitzler, P. (2015). Why the data train needs semantic rails. AI Magazine, 36(1), 5-14.
Khan, W.Z., Ahmed, E., Hakak, S., Yaqoob, I., et al. (2019). Edge computing: A survey. Future Generation Computer Systems, 97, 219–235.
Krämer, B., Stang, M., Nagl, C. & Schäfers, W. (2021). Explainable AI in a Real Estate Context – Exploring the Determinants of Residential Real Estate Values. Available at SSRN: https://ssrn.com/abstract=3989721.
Labee, P., Rasouli, S. & Liao, F. (2022). The implications of Mobility as a Service for urban emissions. Transportation Research Part D: Transport and Environment, 102, 103128.
Lausch, A., Schmidt, A. & Tischendorf, L. (2015). Data mining and linked open data new perspectives for data analysis in environmental research. Ecological Modelling, 295, 5–17.
Mohammadi, M., & Hammink, J. (2015). Standards for Smart Living: A Historical Overview. In J. van Hoof, G. Demeris, E.J.M. Wouters (Eds.), Handbook of Smart Homes, Health Care and Well-Being, 1-14. Springer.
Monekosso, D.N, Remagnino, P. & Kuno. Y. (2009). Intelligent environments: methods, algorithms and applications. In: Monekosso. D., Kuno, Y. (eds.) Advanced information and knowledge processing, 1st ed. Berlin: Springer.
Nunez-Tabalez, J.M., Caridad, J.M., Rey, F.J. (2013). Artificial neural networks for predicting real estate prices. Revista de Metodos Cuantitativos para la Economia y la Empresa, 15 (1), 29-44.
Petrova, E. (2019). AI for BIM-based sustainable building design: Integrating knowledge discovery and semantic data modelling for evidence-based design decision support. Ph.d.-serien for Det Ingeniør- og Naturvidenskabelige Fakultet, Aalborg Universitet: Aalborg Universitetsforlag.
Petrova, E., Pauwels, P., Svidt, K., & Jensen, R.L. (2019). Towards Data-Driven Sustainable Design: Decision Support based on Knowledge Discovery in Disparate Building Data. Architectural Engineering and Design Management, Special Issue on Intelligent Building Paradigms and Data- Driven Models of Innovation, 15 (5), 334-356.
Sacks R., Brilakis I., Pikas E., Xie, H. S. & Girolami, M. (2020). Construction with digital twin information systems. Data-Centric Engineering, 1: e14.
Sowa, J. F. (2008). Handbook of Knowledge Representation. Amsterdam: Elsevier.
Mail the editors